Understanding Labeled Image Datasets: Transforming Business with Data Annotation
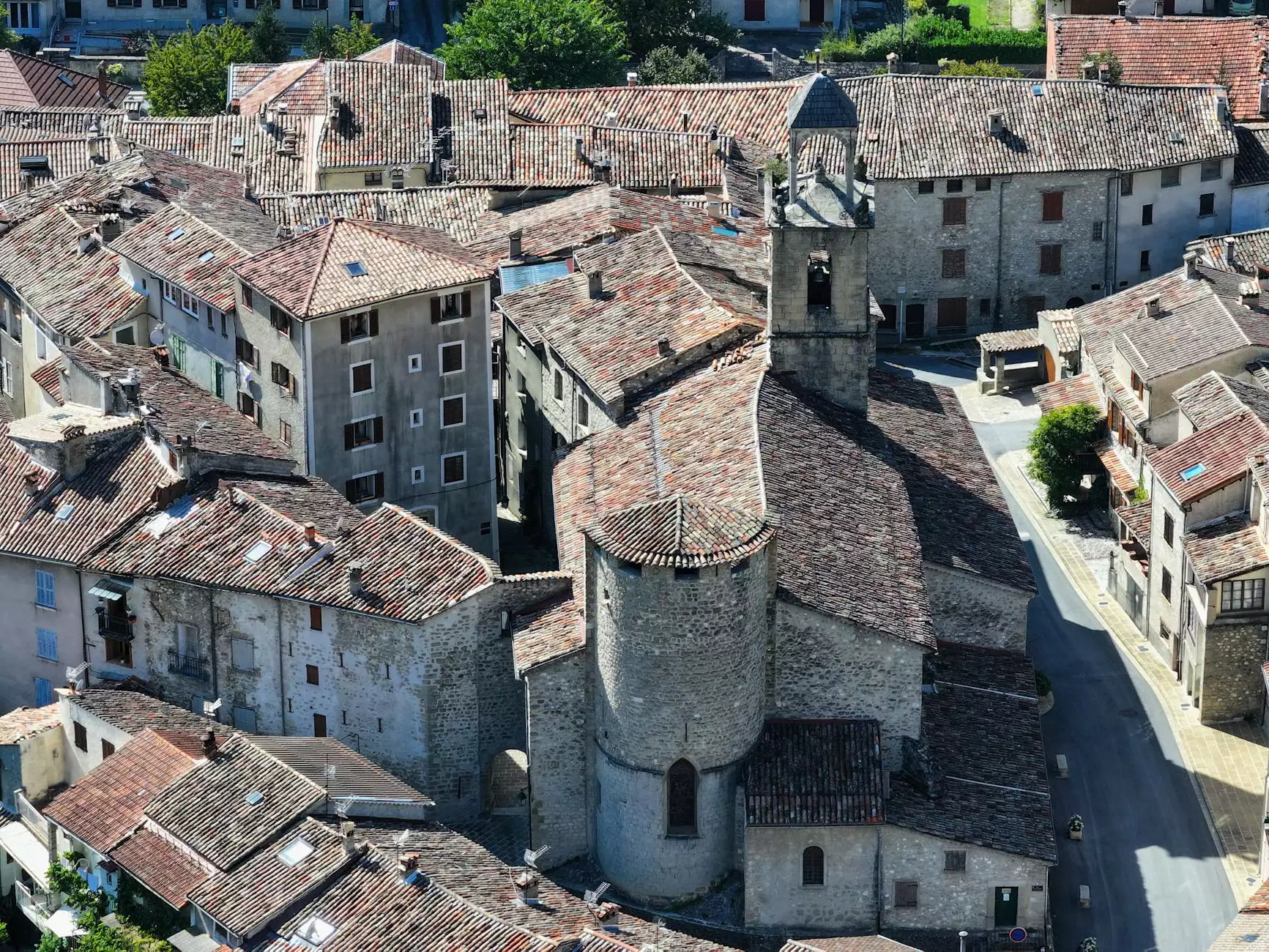
The world of business is rapidly evolving, and one of the most transformative elements in this landscape is data. In particular, labeled image datasets have emerged as a pivotal resource for companies looking to harness the power of artificial intelligence (AI) and machine learning (ML). This article delves into the significance of these datasets, exploring their implications, benefits, and the tools available for effective data annotation.
What are Labeled Image Datasets?
Labeled image datasets consist of images that have been tagged with metadata, providing context and facilitating deep learning in AI applications. These tags can include categorization, objects within the images, and any other relevant information that helps machines interpret visual content. The accuracy and richness of these labels are crucial for training AI models effectively.
The Importance of Data Annotation in Businesses
Data annotation is the bridge that connects raw data with the insights derived from it. The process of labeling data allows businesses to train models that can automate tasks, gain insights, and make predictions. Here are several reasons why data annotation is vital:
- Accuracy and Efficiency: High-quality labeled datasets lead to more accurate predictions and better-performing models.
- Enhanced AI Capabilities: Fine-tuned models powered by labeled datasets enable businesses to automate operations effectively.
- Informed Decision-Making: Annotated datasets provide actionable insights that help businesses strategize and optimize their operations.
Types of Data Annotation
Different projects require different types of data annotation. Here are some common types specifically related to labeled image datasets:
- Image Classification: Images are categorized into classes, making it easier for AI to recognize patterns.
- Object Detection: Specific objects within an image are identified and labeled, providing a bounding box around them for further analysis.
- Semantic Segmentation: Each pixel in the image is tagged with a class label, allowing for detailed level analysis of images.
- Keypoint Annotation: Specific points on an object are marked, which is particularly beneficial in applications like facial recognition or pose detection.
How to Choose the Right Data Annotation Tool
Choosing the right data annotation tool is fundamental for companies looking to create effective labeled image datasets. Here are key factors to consider:
1. Ease of Use
The tool should have an intuitive interface that allows team members to annotate images quickly and accurately without extensive training.
2. Supported Formats
Ensure that the tool supports various image formats and can export labels in the required formats (JSON, XML, CSV, etc.) for integration with AI systems.
3. Collaboration Features
In many businesses, multiple team members will be involved in the annotation process. Choose tools that facilitate easy collaboration and task management among users.
4. Automation Capabilities
With advancements in machine learning, some annotation tools offer semi-automated features, significantly reducing labeling time. Evaluate the extent to which a tool can assist with annotation.
5. Customer Support
A responsive customer support team can assist you when issues arise, ensuring a smooth operation of the annotation process.
Keylabs.ai: A Leader in Data Annotation Solutions
Keylabs.ai is at the forefront of providing comprehensive data annotation solutions that accommodate various business needs. Their platform is designed with features that enhance productivity, quality, and collaboration among teams.
Features of Keylabs.ai’s Data Annotation Platform
- Multi-Format Support: The platform allows for the annotation of various image formats and can easily integrate with existing workflows.
- High Level of Customization: Businesses can create custom labeling schemes that best fit their specific data requirements.
- Quality Assurance Processes: Keylabs.ai incorporates quality control mechanisms to ensure that labeled datasets meet high standards.
- Scalability: Whether you need a few images annotated or millions, Keylabs.ai can scale its services to meet demands.
- Integration Capabilities: The platform can easily connect with existing data pipelines and AI models, enhancing workflow efficiency.
Challenges in Creating Labeled Image Datasets
Despite the clear benefits, creating high-quality labeled image datasets does come with its challenges. Here are some common issues businesses face:
1. Cost
Quality labeling can be resource-intensive, requiring skilled annotators and considerable human effort, leading to increased operational costs.
2. Time-Consuming
The annotation process can take substantial amounts of time, especially when dealing with large datasets, which can delay project timelines.
3. Ensuring Consistency
Maintaining consistency across annotations is vital yet challenging, especially with large teams. Inconsistent labeling can lead to inaccurate models.
Best Practices for Data Annotation
To navigate the challenges of creating labeled image datasets effectively, consider these best practices:
- Define Clear Guidelines: Establish comprehensive annotation guidelines that outline what is expected to ensure consistency.
- Use Experienced Annotators: Skilled annotators can produce better quality labels and reduce the risk of errors.
- Conduct Regular Quality Checks: Implement routine reviews of the labeled data to maintain high standards.
- Incorporate Feedback Mechanisms: Allow annotators to provide feedback on the guidelines and processes to continuously improve efficiency.
The Future of Labeled Image Datasets in Business
As technology evolves, the future of labeled image datasets looks promising. With advancements in AI and machine learning, we can expect:
1. Greater Automation
Future data annotation tools are likely to incorporate more sophisticated AI capabilities, allowing for automated and semi-automated labeling processes.
2. Real-Time Annotation
The emergence of faster computational power will enable real-time annotation, especially for applications like autonomous vehicles and live surveillance systems.
3. Enhanced Collaboration Across Industries
Inter-industry collaborations can lead to the development of enriched datasets that serve multiple sectors, enhancing the quality of machine learning models.
4. Focus on Ethical Considerations
As discussions around data privacy and ethics grow, businesses will need to implement ethical guidelines for data collection and annotation, ensuring responsible use of labeled image datasets.
Conclusion
In conclusion, labeled image datasets are an invaluable asset for businesses looking to leverage data for competitive advantage. By understanding the significance of data annotation and utilizing robust tools and platforms such as Keylabs.ai, companies can unlock the full potential of their data. As we move forward, investing in high-quality labeled datasets will shape the future of AI and machine learning, driving innovation and efficiency in various industries.
For businesses aiming to thrive in this data-driven landscape, prioritizing the creation of labeled image datasets and adopting the right annotation strategies is not just beneficial; it is essential for survival and growth.