Understanding Labelling Tools in Machine Learning
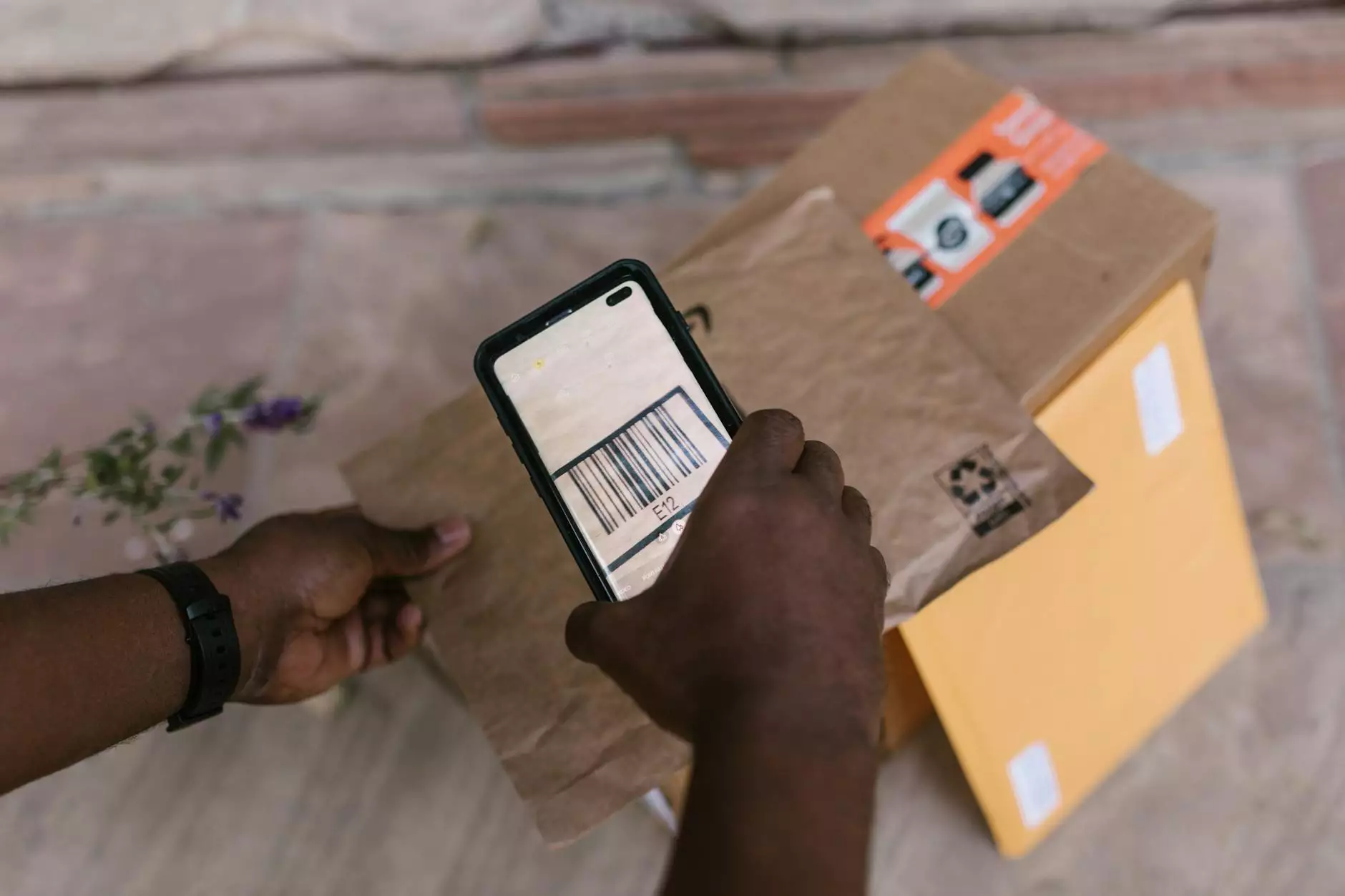
In the fast-paced world of technology, the integration of machine learning into business processes has become increasingly crucial for gaining a competitive edge. As businesses aim to leverage data for actionable insights, the significance of effective data annotation tools cannot be overstated. Specifically, the term labelling tool machine learning emerges as a cornerstone for organizations aiming to develop scalable and efficient machine learning models.
What are Labelling Tools in Machine Learning?
Labelling tools are essential components in the data annotation platform ecosystem, facilitating the classification of various types of data including images, videos, and text. The process of labelling is pivotal in training machine learning algorithms to understand and interpret data accurately. Without properly labelled data, machine learning models cannot achieve their full potential.
As the demand for accurate data continues to grow, the need for advanced labelling solutions has spurred innovation, leading to the development of sophisticated labelling tools powered by machine learning. These tools streamline the annotation process, enhance accuracy, and significantly reduce the time required for data preparation.
Why is Labelling Essential in Machine Learning?
- Model Training: Labelling provides the ground truth needed for supervised learning, which is the backbone of most machine learning algorithms.
- Accuracy: High-quality labelled datasets ensure that models are trained on the best possible data, reducing the risk of errors during the prediction phase.
- Adaptability: Proper labelling enables models to adapt to new data and scenarios, enhancing their applicability across various contexts.
Key Benefits of Using Labelling Tools
The advantages of incorporating labelling tools in your machine learning projects are manifold:
- Efficiency: Automated labelling tools drastically cut down the time taken for manual annotation, allowing teams to focus on other critical tasks.
- Scalability: As your dataset grows, labelling tools can handle massive amounts of data without compromising quality or consistency.
- Collaboration: Many labelling platforms, such as those offered by KeyLabs.AI, support collaborative efforts, enabling teams across different locations to annotate data concurrently.
- Cost-Effectiveness: By reducing the need for extensive manual labour, businesses can significantly lower their operational costs associated with data preparation.
Choosing the Right Labelling Tool for Machine Learning
When selecting a labelling tool machine learning platform, consider the following factors:
1. Compatibility with Data Types
Ensure that the tool can handle various data formats such as images, videos, audio, and text. Flexibility in data input allows for a more inclusive approach to data annotation.
2. User Interface and Experience
A user-friendly interface is important for encouraging team adoption and minimizing the learning curve. The best tools offer intuitive designs that make labelling straightforward and efficient.
3. Automation Features
Look for tools that incorporate AI-driven functionalities to assist in the labelling process. Features like pre-labelling suggestions can speed up the overall annotation workflow.
4. Quality Assurance Mechanisms
Choose a platform that includes built-in quality assurance checks to validate data accuracy and consistency throughout the labelling process.
How KeyLabs.AI Excels in Data Annotation
At KeyLabs.AI, we understand the critical role that effective labelling tools play in the realm of machine learning. Our platform offers comprehensive solutions tailored to meet the demands of modern businesses. Here’s what sets us apart:
Advanced AI-Powered Automation
Our data annotation tool leverages state-of-the-art AI algorithms to assist users in the labelling process, ensuring rapid and accurate results. By minimizing manual intervention, we allow clients to achieve efficiency without sacrificing quality.
Customizable Workflows
We recognize that each project has unique requirements. Our platform allows users to customize workflows to suit specific data types and labelling needs, enabling a tailored approach for optimal results.
Extensive Collaboration Features
Teamwork drives success in data annotation. KeyLabs.AI facilitates seamless collaboration among team members, regardless of their geographical location, making it easier for teams to work together on complex projects.
Case Studies: Successful Implementations of Labelling Tools
Numerous organizations have transformed their data workflows by integrating labelling tools into their machine learning projects. Here are a couple of case studies that illustrate the impact:
Case Study 1: Retail Industry
A major retail brand utilized KeyLabs.AI’s labelling tools to annotate thousands of product images. By implementing our platform, they achieved a 30% reduction in annotation time, enabling faster model development for their recommendation engine. This led to a remarkable 20% increase in customer engagement within just a few months.
Case Study 2: Healthcare Sector
In the healthcare sector, a leading hospital integrated our labelling tools for annotating CT scans for predictive analytics. The automation provided by KeyLabs.AI allowed their team to focus on critical diagnosis work, with a 40% improvement in workflow efficiency and a notable enhancement in diagnostic accuracy.
Future Trends in Labelling Tools and Machine Learning
As we look towards the future, the evolution of labelling tools will continue to play a crucial role in machine learning:
1. Increased Automation
Automation will further enhance the speed and accuracy of data annotation, with machine learning algorithms becoming more adept at identifying patterns and providing pre-labelling suggestions.
2. Ethical AI Considerations
As the use of AI becomes more prevalent, ethical considerations in data labelling processes must be prioritized to ensure fairness and avoid bias in machine learning models.
3. Enhanced User Experiences
The focus will shift towards creating more interactive and user-friendly tools that facilitate easier data labelling, making the process accessible to non-experts.
Conclusion
In the landscape of machine learning, the significance of labelling tool machine learning cannot be understated. Companies that prioritize effective data annotation will find themselves better positioned to harness the power of AI and drive innovation across their business sectors. With KeyLabs.AI, you gain access to cutting-edge data annotation tools that meet the demands of modern data-driven environments.
Investing in robust labelling solutions is not merely a forward-thinking approach but a necessary step for businesses aspiring to leverage machine learning for sustainable growth. Choose wisely and watch how your data transforms into a powerful asset with KeyLabs.AI.