Understanding the Importance of Machine Learning Data Annotation in Modern Business
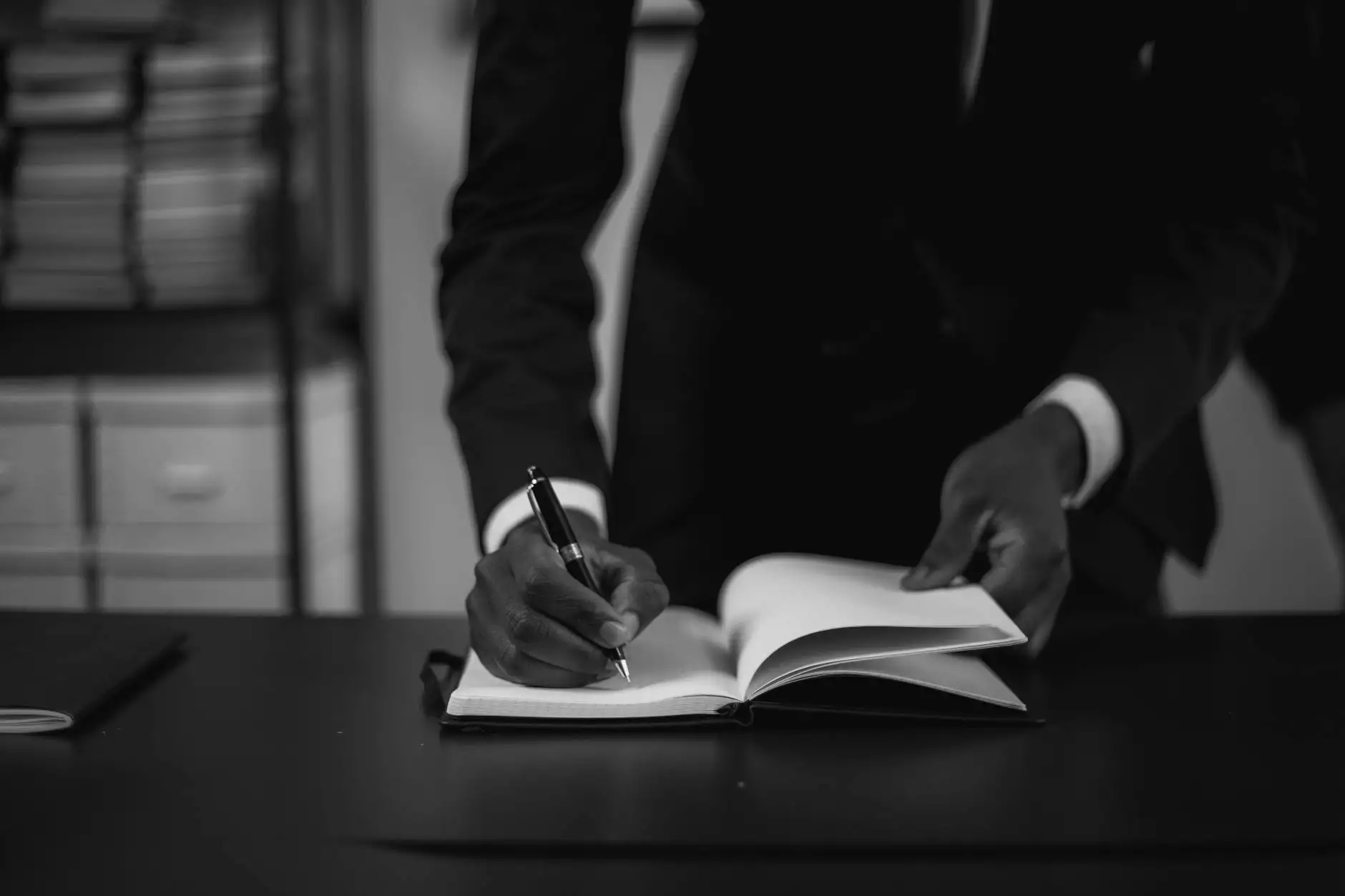
What is Machine Learning Data Annotation?
Machine learning data annotation refers to the process of labeling datasets so that machines can interpret and learn from the data. By annotating data with tags, notes, and other markup, businesses enable AI models to understand context, content, and the relationships between different data points.
This process is crucial in developing systems that can perform tasks ranging from speech recognition to image classification. It ensures that machines are trained on data that has a clear interpretation, paving the way for improved decision-making and performance.
Why is Data Annotation Crucial?
In an era driven by data, the accuracy of machine learning data annotation can significantly influence the quality of artificial intelligence systems deployed across numerous industries. Here’s why it’s crucial:
- Improved Accuracy: Properly annotated data helps models make precise predictions, reducing errors in algorithmic outcomes.
- Efficiency in Training: Annotated datasets accelerate the training process, allowing models to learn faster and more effectively.
- Enhanced Performance: High-quality annotations directly correlate with the performance of machine learning models, impacting overall business functionality.
Applications of Machine Learning Data Annotation
Data annotation plays a vital role in various sectors. Let’s explore some of the key applications:
1. Home Services
In the realm of home services, accurate data annotations can streamline operations. For instance, in predictive maintenance systems, well-annotated historical service records allow AI to forecast equipment failures, thereby improving service efficiency. Companies that leverage machine learning can reduce downtime and enhance customer satisfaction.
2. Keys & Locksmiths
For the locksmith industry, machine learning data annotation can enhance security systems. By annotating images of locks and keys, machine learning models can differentiate between various lock types and security features. This capability assists locksmiths in quickly assessing the type of service needed for a specific lock, improving response times and service quality.
3. Healthcare Sector
In healthcare, data annotation can lead to significant improvements in diagnostics. Medical images, such as X-rays, CT scans, and MRIs, are annotated to assist AI in identifying diseases and conditions, which can enhance patient outcomes through faster and more accurate diagnoses.
The Process of Data Annotation
The data annotation process can broadly fall into several stages:
- Data Collection: Gather relevant datasets from various sources, ensuring a comprehensive pool for annotation.
- Labeling: Apply labels and tags to the collected data; this step might include defining categories for images or transcribing speech to text.
- Quality Assurance: Review the annotated data to ensure consistency and accuracy. This may involve multiple rounds of verification.
- Training AI Models: Use the annotated data to train machine learning algorithms, verifying their performance against expected outcomes.
Challenges in Machine Learning Data Annotation
Despite its importance, data annotation faces several challenges:
- Volume of Data: The sheer amount of data that needs to be annotated can be overwhelming, requiring efficient tools and processes.
- Subjectivity: Depending on the domain, individual annotators may interpret data differently, leading to inconsistencies.
- Quality Control: Ensuring high-quality annotations is time-consuming and may require skilled labor, especially in complex fields like healthcare.
- Cost: The resources needed for data annotation can be significant, impacting project budgets.
The Future of Machine Learning Data Annotation
As technology advances, the landscape of machine learning data annotation is evolving. The integration of automation and AI-driven annotation tools is expected to alleviate many challenges faced today.
These innovations promise:
- Increased Speed: Automated tools can significantly reduce the time it takes to annotate data.
- Better Consistency: AI can help minimize human error and variance in annotation, resulting in more reliable datasets.
- Adaptability: Future systems will be able to learn and adapt, becoming better over time as they process more data.
Conclusion
In conclusion, machine learning data annotation is a critical component in the development of effective AI systems. Whether in home services, keys & locksmiths, or other industries, the ability to accurately label and interpret data can drive significant improvements in service delivery, efficiency, and customer satisfaction. As we look forward, the continued evolution of annotation techniques promises to enhance the capabilities of artificial intelligence, ultimately transforming how businesses operate and serve their customers.
For more insights into the world of data annotation and how it can benefit your business, consider visiting Keymakr.