Introduction About Machine Learning
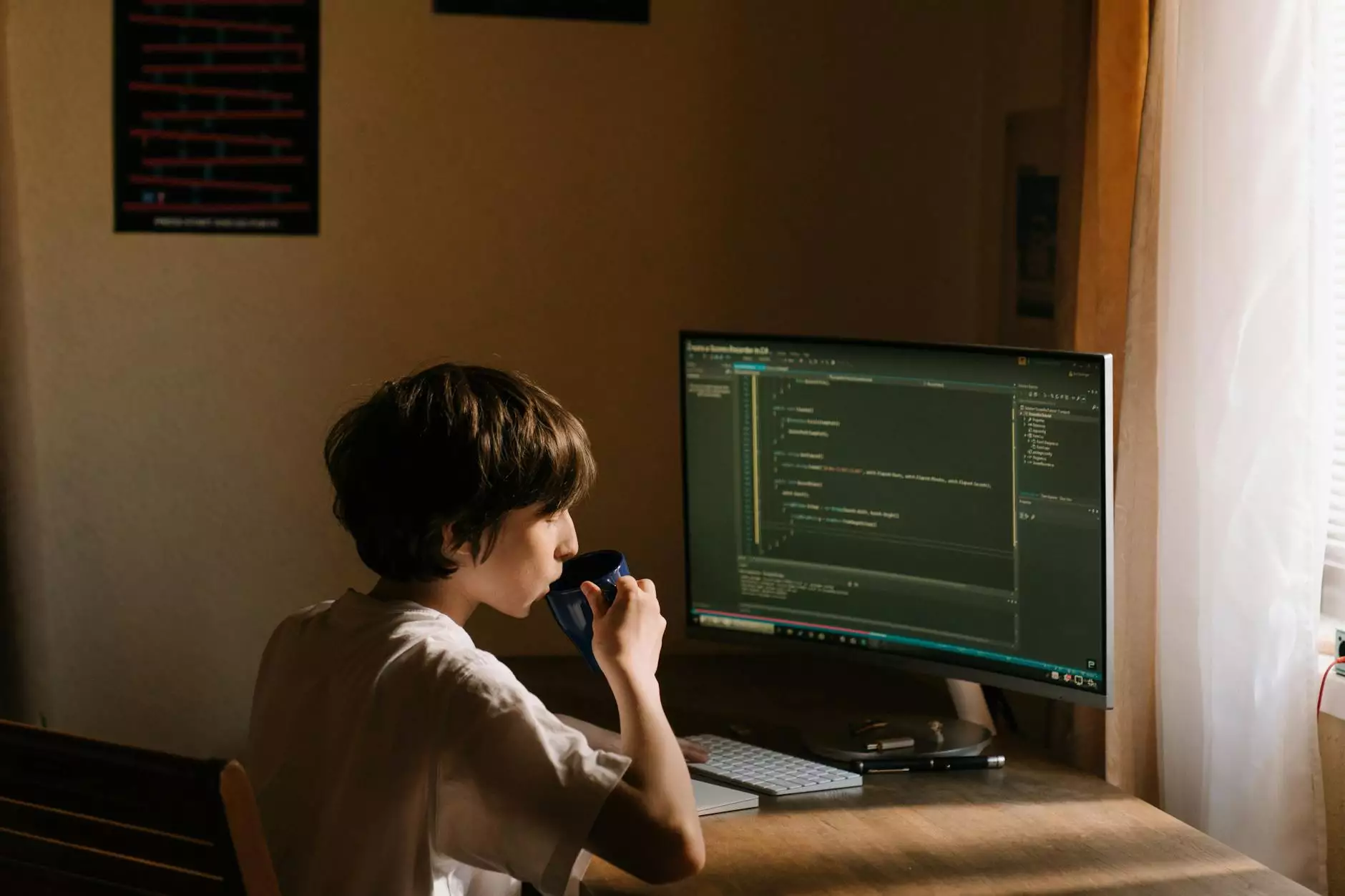
Machine learning has emerged as one of the most transformative technologies of the modern era, revolutionizing various industries by enabling systems to learn from data and improve their performance over time without human intervention. This capability not only enhances operational efficiencies but also drives innovation by uncovering patterns and insights that were previously hidden within vast datasets.
What is Machine Learning?
At its core, machine learning is a subset of artificial intelligence (AI) that focuses on building systems that can learn from and make predictions based on data. It encompasses an array of algorithms aimed at recognizing patterns and making decisions. By leveraging large datasets, machine learning algorithms can improve their accuracy and efficiency over time. This technology has paved the way for advancements in various fields, including finance, healthcare, marketing, and beyond.
The Importance of Machine Learning in Business
In today's competitive landscape, businesses are continuously seeking ways to leverage data for a strategic advantage. Machine learning plays a crucial role in this quest. Here are some key areas where machine learning significantly impacts business:
- Data Analysis and Insights: Machine learning algorithms can analyze complex datasets much faster than traditional methods, identifying trends and providing actionable insights that can inform decision-making.
- Customer Experience: By analyzing customer data, machine learning enables personalized experiences, enhancing customer satisfaction and loyalty. Algorithms can recommend products based on previous purchases, improving user engagement.
- Operational Efficiency: Automating repetitive tasks through machine learning can streamline operations, reduce errors, and save time and resources, ultimately leading to cost reductions.
- Predictive Analytics: Machine learning aids in forecasting trends by predicting future outcomes based on historical data. This is particularly useful in inventory management, sales forecasting, and risk assessment.
- Fraud Detection: Financial institutions utilize machine learning to detect and prevent fraudulent activities by recognizing unusual patterns and behaviors in transaction data.
Types of Machine Learning
Machine learning is broadly classified into several categories based on how the algorithm learns from the data. Understanding these categories is essential for businesses seeking to implement machine learning solutions.
1. Supervised Learning
In supervised learning, algorithms learn from labeled datasets, which means they are provided with input-output pairs. The system learns to map inputs to the outputs, allowing it to make predictions on unseen data. This method is commonly used for classification and regression tasks, such as spam detection and sales forecasting.
2. Unsupervised Learning
Unsupervised learning algorithms work with unlabeled data, finding hidden patterns or intrinsic structures within the data. This type is particularly useful for clustering customer segments or market analysis, where the system groups data points based on similarities without predefined labels.
3. Reinforcement Learning
Reinforcement learning focuses on training algorithms through a system of rewards and penalties. The algorithm learns by performing actions in an environment and receiving feedback, optimizing its strategy over time. This approach is often applied in robotics, gaming, and automation.
Applications of Machine Learning in Various Industries
Machine learning's versatility allows it to be applied across numerous industries, creating numerous opportunities for businesses to innovate and enhance their operations.
1. Healthcare
In healthcare, machine learning assists in diagnosing diseases, personalizing treatment plans, and managing patient data. Algorithms can analyze medical images faster and more accurately than humans, leading to early detection of conditions such as cancer.
2. Finance
Financial institutions leverage machine learning for risk management, fraud detection, and algorithmic trading. By analyzing spending patterns and transaction histories, machine learning systems can identify anomalies indicative of fraud.
3. E-commerce
In the e-commerce sector, personalized recommendations, price optimization, and inventory management are some of the key applications of machine learning. Systems analyze user behavior to suggest products, improving conversion rates and customer satisfaction.
4. Manufacturing
Machine learning enhances manufacturing processes by predicting equipment failures, optimizing supply chain management, and improving quality control. Predictive maintenance algorithms can foresee potential breakdowns, minimizing downtime.
5. Marketing
Marketing teams use machine learning to analyze consumer behavior, segment target audiences, and optimize advertising spend. The ability to predict campaign success and customer preferences translates into more effective marketing strategies.
Challenges and Considerations
While the potential of machine learning is immense, there are several challenges that businesses must address when implementing these technologies:
- Data Quality: For machine learning systems to be effective, they require high-quality, clean data. Inaccuracies or biases in data can lead to faulty predictions and undermine trust in the system.
- Model Interpretability: Many machine learning models, especially deep learning, operate as "black boxes," making it difficult to understand their decision-making processes. This lack of transparency can pose risks, especially in sensitive areas like healthcare and finance.
- Integration with Existing Systems: Businesses often face challenges integrating machine learning solutions with their existing infrastructure. A well-thought-out implementation strategy is necessary for success.
- Ethical Considerations: The potential for bias in algorithms raises ethical concerns regarding data privacy, discrimination, and accountability. Businesses must develop responsible AI practices to mitigate these issues.
Future Trends in Machine Learning
The future of machine learning looks promising, with ongoing advancements shaping how businesses utilize this technology:
1. Explainable AI
As organizations demand more transparency from AI systems, explainable AI (XAI) is becoming crucial. This approach aims to make machine learning models more interpretable, allowing users to understand the reasoning behind predictions.
2. Autonomous Systems
Autonomous systems leveraging machine learning are expected to grow, particularly in areas like self-driving cars and smart drones. As safety and reliability improve, these systems will likely see wider acceptance in various industries.
3. Increased Use of Edge Computing
With the proliferation of IoT devices, machine learning is increasingly being executed on the edge, closer to where data is generated. This reduces latency and bandwidth requirements, enabling real-time data processing and decision-making.
4. Enhanced Natural Language Processing
Advancements in natural language processing (NLP) will further enhance how machines understand and interact with human language, leading to more sophisticated chatbots, virtual assistants, and automated translation services.
5. Democratization of Machine Learning
As machine learning platforms become more accessible, businesses of all sizes can harness the power of AI without requiring extensive data science expertise. This democratization will lead to greater innovation and use of machine learning across various sectors.
Conclusion
Machine learning is no longer just a buzzword; it is a fundamental component of modern business strategy. By embracing machine learning, organizations can unlock significant advantages, enhance operational efficiency, and create innovative solutions that better serve their customers. As technology continues to evolve, the potential applications and benefits of machine learning will only expand, making it an essential area of focus for any forward-thinking business.
In conclusion, whether you're a budding entrepreneur or a seasoned business leader, understanding and implementing machine learning strategies could be the key to unlocking new opportunities and staying ahead in today’s data-driven world.
introduction about machine learning